Robust Production - Inventory¶
Consider the problem of minimizing the costs associated with inventory management while ensuring that customer demand is met consistently over a planning horizon. This approach addresses the inherant uncertainty in product demands, making it a robust optimization problem.
Taking the example introduced in Ben-Tal et al. (2004) [1], let us consider a single product inventory system which is comprised of a single warehouse and \(I\) identical factories. The planning horizon is \(T\) periods. At time period \(t\), let:
\(d_t\) denote the demand of the product at each that is uncertain.
\(v_t\) denote the amount of product in the warehouse at the beginning of the time period.
\(p_t\in {\bf R}^I\) denote the amount of product to be produced during period \(t\) by each factory.
\(c_t \in {\bf R}^I\) denote the cost of producing a unit of product at each factory at time period \(t\).
\(p^{\rm max}\) denote the maximal production capacity for each factory at time period \(t\).
\(q^{\rm max}\) denote the maximal cumulative production capacity for each factory.
\(v^{\rm min}\) and \(v^{\rm max}\) denote the minimal and maximal storage capacity of the warehouse, respectively.
With this information, we can write the inventory problem as a robust linear program
[1]:
import numpy as np
import cvxpy as cp
import lropt
import matplotlib.pyplot as plt
Next, we consider \(I\) = 3 factories producing a product in one warehouse. The time horizon \(T\) is 24 time periods. The maximal production capacity of each one of the factories at each two-weeks period is \(P(t) = 567\), and the integral production capacity of each one of the factories for a year is \(Q = 13600\). The inventory at the warehouse should not be less then \(300\) units, and cannot exceed \(10000\) units. Initially, the inventory sits at \(500\) units.
[2]:
np.random.seed(1)
T = 24 #number of periods
I = 3 #number of factories
v_min = 300
v_max = 10000
q_max = 13600
p_max = 567
v_init = 500
alphas = np.array([1, 1.5, 2])
The production cost for at period \(t\) for each factory \(i\) is given by:
where \(\alpha = (1, 1.5, 2)\). The demand is uncertain and seasonal, reaching its peak in winter, with the following pattern,
To handle this uncertainty, we adopt the Polyhedral uncertainty set. This uncertainty set is defined by:
The following code defines these parameters for the problem.
[3]:
def setup_parameters(proportion):
# Check if you can use t_values here as well instead of the list comprehension
t_values = np.arange(1, T + 1)
c = [(alphas * (1 + 0.5 * np.sin(np.pi * (t - 1) / (T * 0.5)))).flatten() for t in range(1, T + 1)]
c = np.array(c).T
d_star = 1000 * (1 + 0.5 * np.sin(np.pi * (t_values - 1) / (T * 0.5)))
lhs = np.concatenate((np.eye(T), -np.eye(T)), axis=0)
rhs_upper = (1 + proportion) * d_star
rhs_lower = (-1 + proportion) * d_star
rhs = np.hstack((rhs_upper, rhs_lower))
d = lropt.UncertainParameter(T, uncertainty_set=lropt.Polyhedral(lhs=lhs, rhs=rhs))
return c, d, rhs_upper, rhs_lower, d_star
Next, we create a function to define the constraints of the function.
This is a function that solves the problem and returns the optimal value.
[4]:
def solve_problem(d):
p = cp.Variable((I, T), nonneg=True)
constraints = [
p <= p_max,
0 <= p,
cp.sum(p, axis=1) <= q_max
]
for i in range(1, T + 1):
constraints.append(v_min <= cp.sum(cp.sum(cp.sum(p, axis=0)[:i])) - cp.sum(d[:i]) + v_init)
constraints.append(cp.sum(cp.sum(cp.sum(p, axis=0)[:i])) - cp.sum(d[:i]) + v_init <= v_max)
objective = cp.Minimize(cp.sum(cp.multiply(c, p)))
prob = lropt.RobustProblem(objective, constraints)
prob.solve()
return prob.objective.value, p.value
The following code calculates the robust optimal level of inventory over time.
[5]:
def calculate_inventory_levels(p, rhs_upper, d_star, rhs_lower):
low_values = []
mid_values = []
high_values = []
random_trajectory = []
for i in range(1, T + 1):
low = (cp.sum(cp.sum(p, 0)[:i]) - cp.sum(rhs_upper[:i]) + v_init).value
mid = (cp.sum(cp.sum(p, 0)[:i]) - cp.sum(d_star[:i]) + v_init).value
high = (cp.sum(cp.sum(p, 0)[:i]) - cp.sum(-rhs_lower[:i]) + v_init).value
# TODO: create random trajectory
low_values.append(low)
mid_values.append(mid)
high_values.append(high)
random_trajectory.append(np.random.uniform(low, high))
return low_values, mid_values, high_values, random_trajectory
Finally, we define a function to plot the inventory level over time for a given value of proportion.
[6]:
def plot_inventory_levels(ax, low_values, mid_values, high_values, random_trajectory, rho):
ax.plot(range(1, T + 1), low_values, label='Disturbance Lower Bound', color='blue', linestyle='--')
ax.plot(range(1, T + 1), mid_values, label='Nominal Disturbance', color='green', linestyle='-')
ax.plot(range(1, T + 1), high_values, label='Disturbance Upper Bound', color='red', linestyle='--')
ax.plot(range(1, T + 1), random_trajectory, label='Random Trajectory', color='lightgreen', linestyle='--')
ax.set_xlabel('Time Period')
ax.set_ylabel('Inventory Level')
ax.set_title(f'Inventory Level Over Time\nrho = {rho}')
ax.legend()
ax.grid(True)
[7]:
fig, axs = plt.subplots(1, 2, figsize=(18, 8))
rho_values = [0.1, 0.2]
for i, rho in enumerate(rho_values):
c, d, rhs_upper, rhs_lower, d_star = setup_parameters(rho)
optimal_value, p_value = solve_problem(d)
print(f"rho = {rho}: The robust optimal value is {optimal_value:.3E}")
low_values, mid_values, high_values, random_traj = calculate_inventory_levels(p_value, rhs_upper, d_star, rhs_lower)
plot_inventory_levels(axs[i], low_values, mid_values, high_values,random_traj, rho)
plt.tight_layout()
plt.show()
/Users/mj5676/Desktop/miniconda3/envs/lropt_v3/lib/python3.12/site-packages/cvxpy/utilities/torch_utils.py:61: UserWarning: torch.sparse.SparseTensor(indices, values, shape, *, device=) is deprecated. Please use torch.sparse_coo_tensor(indices, values, shape, dtype=, device=). (Triggered internally at /Users/runner/work/pytorch/pytorch/pytorch/torch/csrc/utils/tensor_new.cpp:643.)
return torch.sparse.FloatTensor(i, v, torch.Size(value_coo.shape)).to(dtype)
rho = 0.1: The robust optimal value is 3.830E+04
rho = 0.2: The robust optimal value is 4.367E+04
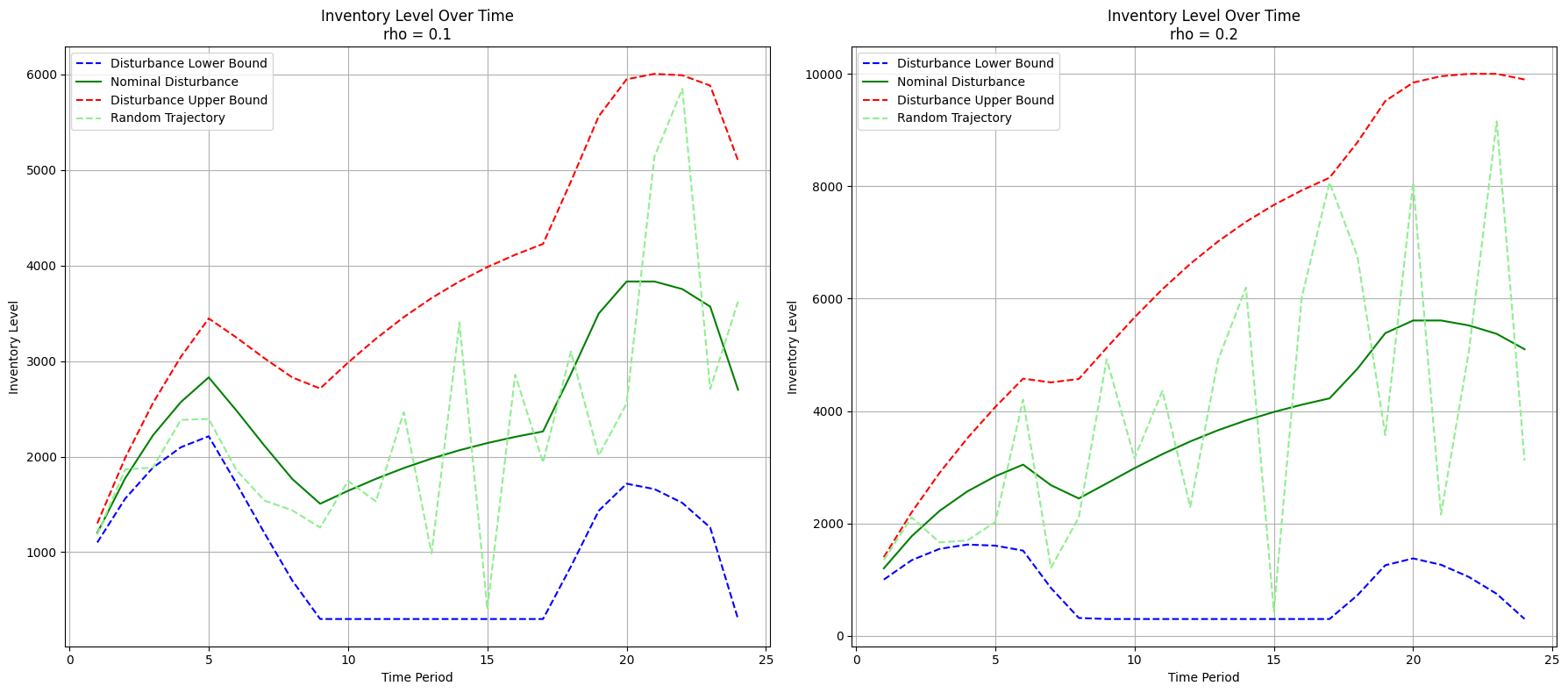
References¶
Ben-Tal, Aharon, Alexander Goryashko, Elana Guslitzer, and Arkadi Nemirovski. 2004. Adjustable robust solutions of uncertain linear programs. Mathematical Programming 99(2) 351-376. - add a clickable link